Machine Learning (ML) has emerged as a key player in modern technology, allowing computers to learn from data and make intelligent decisions without specific programming for each task. Whether it’s helping predict diseases, enabling self-driving cars, or personalizing our online experience, ML has quickly become an essential part of our lives. Let’s break down how machine learning works, its various types, and the profound impact it’s making across industries.
What is Machine Learning?
Machine Learning is a subset of Artificial Intelligence (AI) that enables systems to learn and improve from experience. Unlike traditional programming, where we give explicit instructions, ML models learn patterns from data and adapt based on new information. This ability to self-improve and adapt makes ML a powerful tool for handling complex, data-driven tasks.
Key Types of Machine Learning
There are several different approaches within ML, each suited to distinct tasks and challenges:
1. Supervised Learning: Learning with Labels
In supervised learning, algorithms are trained on labeled datasets, meaning each example includes an input and the correct output. The model uses these examples to learn how to map inputs to outputs, which makes supervised learning ideal for tasks like classification (e.g., spam vs. non-spam emails) and regression (e.g., predicting house prices based on features).
2. Unsupervised Learning: Discovering Patterns
In contrast, unsupervised learning works without labeled data. Here, the system tries to understand the underlying structure within a dataset. It’s especially useful for clustering (e.g., grouping customers with similar purchasing habits) and association (e.g., identifying frequently bought items together), which help reveal patterns in data without predefined categories.
3. Reinforcement Learning: Learning through Rewards
Reinforcement learning takes a different approach, allowing algorithms to learn through a system of rewards and penalties based on actions taken in a given environment. This method is widely used in dynamic applications, like training robots to navigate spaces or creating AI that can play video games, as it encourages the model to maximize positive outcomes over time.
Real-World Applications of Machine Learning
Machine learning has found applications across numerous industries, transforming how organizations solve complex challenges and offer personalized services:
- Healthcare: ML models are used to predict patient outcomes, diagnose diseases early, and personalize treatment plans, helping improve patient care and efficiency.
- Finance: From fraud detection and credit scoring to algorithmic trading, ML empowers financial institutions to make faster, data-driven decisions while enhancing security and service quality.
- Retail: ML enables personalized product recommendations, helps optimize inventory management, and improves customer experiences by analyzing buying patterns and preferences.
- Transportation: Machine learning is integral to autonomous vehicle technology, helping vehicles navigate roads safely. It also supports predictive maintenance, minimizing disruptions and extending equipment life.
Challenges and Considerations in Machine Learning
While machine learning offers powerful solutions, it comes with its own set of challenges:
- Data Quality: The accuracy of an ML model depends heavily on the quality of the training data. Poor-quality data leads to poor-quality predictions, which can undermine the model’s effectiveness.
- Bias and Fairness: ML models can unintentionally learn and amplify biases present in the training data, leading to unfair or discriminatory results. Addressing bias is crucial to ensure that ML applications are equitable.
- Interpretability: Many advanced ML models, especially deep learning models, are complex and often seen as “black boxes.” Ensuring transparency and understanding in how these models make decisions remains a major focus in ML research.
The Future of Machine Learning: Driving Innovation
As data continues to grow exponentially, machine learning is expected to become even more central to technological innovation. Research is ongoing to create more efficient, fair, and interpretable models, ensuring ML’s positive impact across sectors and society at large.
Embracing the Power of Machine Learning
Understanding the potential and limitations of machine learning is essential in today’s tech-driven world. As ML continues to evolve, it opens new doors for advancements in AI, paving the way for smarter, more adaptive technologies that can tackle complex, real-world challenges.
Read Also:
- The Evolution from Data to Big Data: A Journey Through Decades of Innovation
- Understanding Artificial Intelligence: A Human-Centric Overview
- Addressing AI Risks: Achieving the AI Risk Management Professional Certification
- Mastering Scaled Scrum: Earning the Scaled Scrum Professional Certification
- Strengthening Agile Leadership: Achieving the Scrum Master Professional Certificate
- Advancing My Expertise in AI: Earning the CAIEC Certification
- Achieving the CAIPC Certification: Advancing My AI Expertise
- Foundational Python Data Structures for AI-Driven Applications
Subscribe to the GnoelixiAI Hub newsletter on LinkedIn and stay up to date with the latest AI news and trends.
Subscribe to my YouTube channel.
Reference: aartemiou.com (https://www.aartemiou.com)
© Artemakis Artemiou
Rate this article:
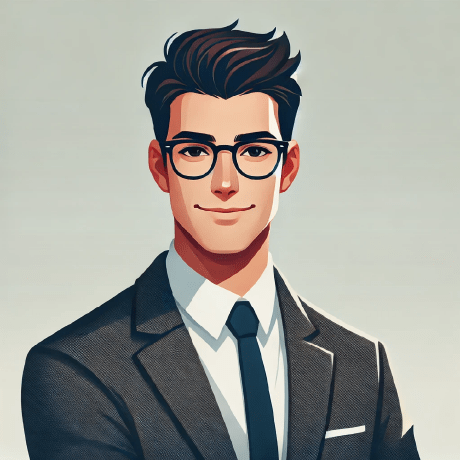
Artemakis Artemiou is a seasoned Senior Database and AI/Automation Architect with over 20 years of expertise in the IT industry. As a Certified Database, Cloud, and AI professional, he has been recognized as a thought leader, earning the prestigious Microsoft Data Platform MVP title for nine consecutive years (2009-2018). Driven by a passion for simplifying complex topics, Artemakis shares his expertise through articles, online courses, and speaking engagements. He empowers professionals around the globe to excel in Databases, Cloud, AI, Automation, and Software Development. Committed to innovation and education, Artemakis strives to make technology accessible and impactful for everyone.